Deep Neural Network to Find Hidden Turbulent Motion on the Sun - New Development in Research for Solar and Plasma Turbulence -
2022.2.25National Institutes of Natural Sciences, National Astronomical Observatory of Japan (NAOJ)
National Institutes of Natural Sciences, National Institute for Fusion Science (NIFS)
The Graduate University for Advanced Studies (SOKENDAI)
Research Results (Press Release)Abstract
A research group led by the National Astronomical Observatory of Japan (NAOJ), the National Institute for Fusion Science (NIFS), and the Graduate University for Advanced Studies (SOKENDAI) has succeeded in constructing a new deep learning model that infers hidden turbulent motion from image data of the solar surface. Using a number of data from several numerical simulations that reproduce solar convection, a neural network was trained to learn the correlation between the horizontal motion of turbulence, which is diffcult to observe directly, and the vertical motion of turbulence and surface temperature, which are observable. As a result, a new analysis method to infer the horizontal motion of turbulence from the image data of vertical motion and surface temperature has been realized. Furthermore, a new method for analyzing the inference accuracy was developed, and it was found a rapid change of the inference accuracy for structures smaller than the typical size of generated convection cells. By clarifying effectiveness of the method in analyzing actual solar turbulence data and the current achievement of the inference accuracy, we have obtained a clue for further improvement of the method in the future. This research was promoted within the framework of the NINS cross-disciplinary study for Transport, and Heating Dynamics in Laboratory and Solar/Astrophysical Plasmas: SoLaBo-X. A new development is underway to apply the deep learning model developed in this research to the study of turbulence fluctuation in fusion plasmas.
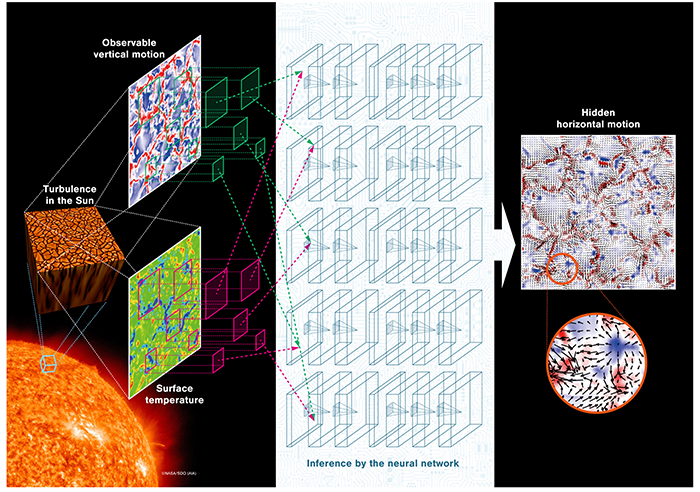
Figure 1: Conceptual diagram of this work. Given the observable surface temperature and vertical motion of turbulent flows on the solar surface, the neural network is able to infer the horizontal motion (Credit: NAOJ).
Main text
On the surface of a star such as the Sun, thermal convection causes strong turbulent flows. The patterns created by convection on the solar surface are called granulation (Note-1). Turbulence on the solar surface is thought to play a role in supplying heating energy to the corona in the Sun's outer atmosphere by amplifying and shaking the magnetic field, and research is carried out to elucidate the mechanism of this phenomenon. Therefore, it is necessary to measure the velocity of the turbulence and its spatial distribution. The vertical motion can be measured using the Doppler effect (Note-2), but the horizontal motion cannot be measured directly. Correlation tracking methods that have been used in the past research are limited to an inference of convective motion on a spatial scale equal to or larger than the size of granulation. Inference of horizontal motion using deep learning has also been attempted, but the cause of inadequate accuracy of the method is not well understood.
A research group led by Ryohtaroh T. Ishikawa (Graduate University for Advanced Studies and National Astronomical Observatory of Japan), Motoki Nakata (National Institute for Fusion Science), Yukio Katsukawa (National Astronomical Observatory of Japan), Youhei Masada (Aichi University of Education), and Tino L. Riethmüller (Max Planck Institute for Solar System Research) utilized numerical simulations for solar thermal convection. Using a number of data from three sets of numerical simulations, the research group developed a deep neural network to correlate the observable structure of temperature and vertical motion on the solar surface with the horizontal motion that is diffcult to observe. As a result, a new analysis method that can rapidly infer the horizontal motion of turbulence, which is diffcult to observe, from image data of vertical motion and surface temperature has been realized. The new feature of this research is that we constructed a multi-scale convolutional neural network that takes into account the characteristics of turbulence with various spatial scales, and also developed a method to analyze the inference accuracy as a function of spatial scales. Thanks to the approach, we found that turbulence structures smaller than the typical size of generated convection limit the accuracy of horizontal motion estimation. By clarifying effectiveness of the method in analyzing actual solar turbulence data and the current achievement of the inference accuracy, we have obtained a clue for further improvement of the method in the future.
Exploring a turbulence structure is a common issue not only in solar research but also in plasma physics and fusion science, and other research for complex flows. This research was also promoted within the framework of the NINS cross-disciplinary study for Transport, and Heating Dynamics in Laboratory and Solar/Astrophysical Plasmas: SoLaBo-X. A new development is underway to apply the deep learning model developed in this research to the study of turbulence fluctuation in fusion plasmas.
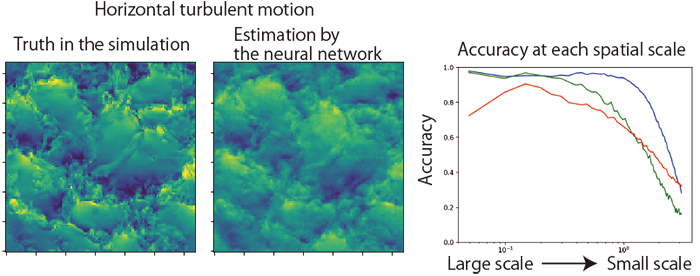
Figure 2: (Left) From left to right, the horizontal motion of turbulence (numerical simulation) and the horizontal motion inferred by deep learning. The light and dark areas correspond to the upward and downward flow on the paper, respectively. (Right) Estimation accuracy at different spatial scales. The different colors of the lines indicate the estimation accuracy for the horizontal motion of turbulent flows with different characteristics (Credit: NAOJ).
Paper
"Multi-Scale Deep Learning for Estimating Horizontal Velocity Fields on the Solar Surface"
Authors:Ryohtaroh T. Ishikawa (NAOJ, SOKENDAI), Motoki Nakata (NIFS), Yukio Katsukawa (NAOJ),Youhei Masada (AUE), and Tino L. Riethmüller (MPS)
Journal: Astronomy & Astrophysics, Volume 658, A142 (publised online on February 16, 2022)
DOI: 10.1051/0004-6361/202141743
Acknowledgments for Research Grants
- FY2019-2021 Grant-in-Aid for JSPS Fellows 19J20294 (PI: Ryohtaroh T. Ishikawa)
- FY2019-2021 NINS cross-disciplinary study for Transport, and Heating Dynamics in Laboratory and Solar/Astrophysical Plasmas: SoLaBo-X, 01321802, 01311904 (PI: Motoki Nakata)
- FY2018-2022 Grant-in-Aid for Scientifc Research(S) (PI: Yukio Katsukawa)
- FY2020-2023 Grant-in-Aid for Fostering Joint International Research (B) 20KK0072 (PI: Shin Toriumi)
Note
- Granulation
Cellular patterns, found on the solar surface, are about 1,000 km in size and have an average lifetime of about 10 minutes, and reflect the temperature structure caused by convective motion. - Doppler effect
By measuring the change in wavelength of a spectral line emitted by the atmosphere, we can tell whether it is approaching or moving away, and its velocity.
- Deep Neural Network to Find Hidden Turbulent Motion on the Sun (NAOJ)
- Deep Neural Network to Find Hidden Turbulent Motion on the Sun (Solar Science Observatory)
- Deep Neural Network to Find Hidden Turbulent Motion on the Sun (SOKENDAI)